- Ann Kellett, PhD
- Public Health
New method improves survival analysis power in clinical and epidemiological studies
Innovative statistical method helps determine ideal threshold times in restricted mean survival time analyses
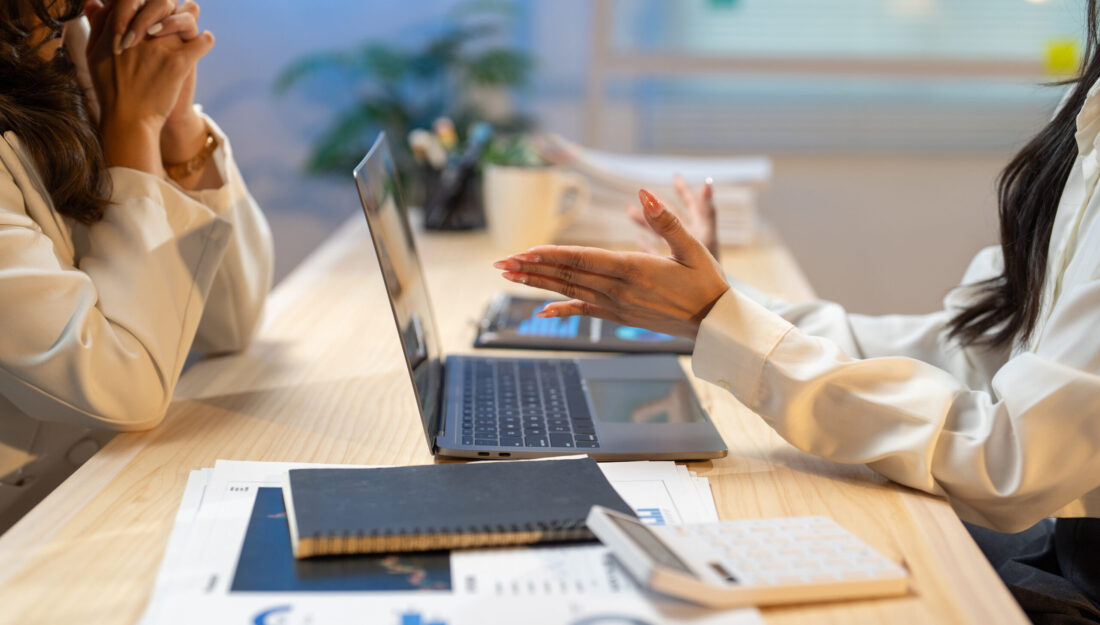
The new method uses the reduced piecewise exponential model from mathematics. (Adobe Stock)
The restricted mean survival time (RMST) analysis technique was introduced in health care research about 25 years ago and since then has become widely used in economics, engineering, business and other professions.
In clinical settings, RMST is useful because it is a straightforward way to understand the average survival time—the length of time patients live after diagnosis or treatment and the factors that affect that time—within a specified timeframe.
In addition, unlike Cox regression models and other popular models, estimations and comparisons made using RMST do not rely on the proportional hazard assumption that the likelihood of an event happening will be constant over time.
“But there’s a catch: RMST can test differences in a treatment’s effect between groups from baseline to a time point—the threshold—but identifying the ideal threshold in clinical and epidemiological studies is difficult,” said Gang Han, PhD, biostatistics professor in the Texas A&M University School of Public Health. “This leads to results that are less statistically powerful than they could be.”
To address this challenge, Han and colleagues in academia and industry have developed a new method that uses an existing mathematical tool—the reduced piecewise exponential model—to determine the ideal or optimal threshold time in the restricted mean survival time analysis when studying two groups.
“This is especially important in medical studies, since the probability of a specific event happening can change over the different stages of treatment,” said Matthew Lee Smith, PhD, a health behavior professor in the Texas A&M School of Public Health, who was involved in this research.
To determine the optimal threshold, the team calculated a threshold time from significant changepoint(s) in hazard rates and compared what they found with the largest possible threshold time.
Their research paper, published in the American Journal of Epidemiology, showed the benefits of the proposed method in multiple simulation studies and two real examples, a clinical study and an epidemiology study.
They used the new method to measure Type 1 error rates and statistical power in simulations in which the hazard rate was constant for one group and was changed for another group. They compared the groups using the standard logrank test and their new model.
“Our model performed the best,” said Marcia G. Ory, PhD, Regents and Distinguished Professor in the School of Public Health who researches evidence-based prevention methods. “That also was the case when we applied it to two real-world scenarios.”
For both scenarios, traditional statistical analysis methods revealed no notable differences between two treatments. When the new model was applied, however, the results for each scenario found that one treatment was clearly superior.
The first scenario compared two treatments over seven months for patients with non-small-cell lung cancer who had lower levels of a key biomarker. The second used a standard assessment to measure the time to decline of people with mild dementia who lived with caregivers compared to those who did not live with caregivers.
“These results are promising, and more research is needed that compares more than two groups and that uses multiple covariates, such as participants’ age, ethnicity and socioeconomic status,” Han said. “Still, based on these early results, we believe this method could be more powerful than all existing comparisons for two groups in the analysis of time-to-event outcomes.”
Others involved in the study were Department of Epidemiology and Biostatistics doctoral student Laura Hopkins, Raymond Carroll, PhD, Distinguished Professor in the Texas A&M Department of Statistics and external collaborators from Eli Lilly and Company, and the H. Lee Moffitt Cancer Center & Research Institute.
Media contact: media@tamu.edu